Taking a Deep Dive into Climate Data
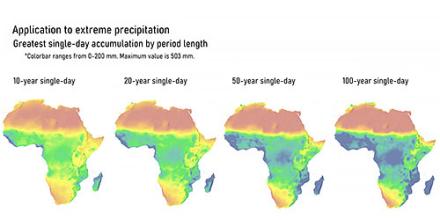
Our climate is ever-changing, and the stress these conditions are placing on our crops is tremendous. Drought, increasing rainfall, and extreme weather conditions are adversely affecting the health of our soil as well as the hydrological cycle. In order to study current and future weather patterns in different regions across the globe, researchers need accurate modeling tools based on atmospheric data.
Accurately modeling variables such as precipitation and drought requires long-term, high-resolution, and high-quality data for a number of different variables. However, datasets that meet these requirements are often not available, especially across large swaths of land in the Global South, such as Africa and South America.
“Ground stations providing long-term climate observations are largely concentrated in Europe, Australia, and North America, and this hampers our ability to model current and future evolution of critical climate parameters across large areas of land,” said Dr. Andrew Fullhart, post-doc at ARS’s Southwest Watershed Research Center in Tucson, AZ. Fullhart is also a member of the Long-term Agroecosystem Research network.
Researchers currently apply transformations to popular global datasets to estimate an average variable value at a given single (point-scale) location. One such transformation is called statistical downscaling. The popular weather generator CLImate GENerator (CLIGEN) can be used for statistical downscaling of global data in areas where primary data sources are scarce or non-existent.
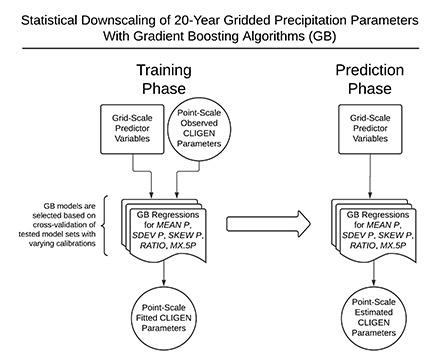
However, statistical downscaling can result in significant modeling uncertainties because regional and local climate conditions can be complex and variable. What is needed is a strong climatic baseline to use in conjunction with CLIGEN to more accurately predict future weather patterns. Recently, Fullhart and his colleagues developed a CLIGEN gridded dataset, yielding 20-year average point-scale data across Africa and South America. Their approach made use of powerful machine learning-techniques that analyze 21 variables, including mean precipitation, annual rainfall, surface air pressure, latitude, and elevation, along with a variety of statistical measures.
The grid created by Fullhart includes 40,936 points in Africa and 24,588 points in South America based on data from the past two decades. Fullhart utilized several data sources to compile this gridded dataset, including satellite-based remote sensing datasets and climate model reanalyzes – creating a comprehensive record of weather dynamics during the 21st century. Fullhart also collected directly observed precipitation data from two global ground-based networks, covering over 11,000 stations.
Fullhart and his team found that their gridded dataset provided accurate monthly, daily, and sub-daily point-scale values for different variables across different land masses.
“Using the point-scale data downscaled by CLIGEN, a number of agricultural and hydrological models can be applied in the highly heterogenous spatial distribution of rainfall around the globe, including in places like Africa, from the very low precipitation of the Sahara, Sahel, and Kalahari regions to the very high precipitation of the Congo Basin,” he said.
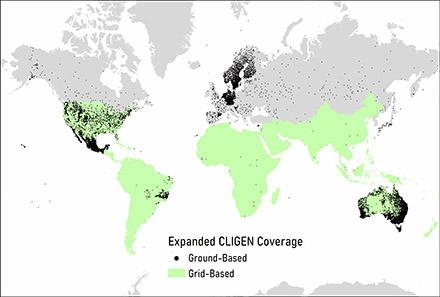
A common application of CLIGEN is to drive model simulations of soil erosion caused by rainfall and runoff. Efforts were made to verify the accuracy of extreme precipitation produced by CLIGEN, and subsequently, erosion predictions during extreme rainfall may be better informed. Specifically, it was found that certain cases of extreme precipitation are overestimated by CLIGEN, but overall, CLIGEN works well for its relative simplicity and minimal requisite inputs.
“With more accurate and detailed climate model reanalyzes and satellite data, along with improved machine learning, we will be able to better model extreme weather events and predict their impact on our lands,” he said. “For the purposes of risk-assessment, CLIGEN can help produce arbitrarily long records, which is beneficial for understanding long-term averages and infrequent extreme events and testing novel hydrological hypotheses.”
The key is coverage, and when considered together with all known CLIGEN grids, coverage is now available for the majority of ice-free global land masses. With these improvements to climate modeling capabilities, land managers can more effectively manage and mitigate global climate change and its consequences. - By Todd Silver, ARS’s Office of Communications
You May Also Like: